Machine learning has dramatically transformed search algorithms over the last decade, reshaping the way information is retrieved. This transformation has enhanced search accuracy, personalization, and user experience across various platforms. At Metrics Rule in Vancouver, we focus on how these advancements in algorithms influence SEO strategies and digital marketing practices. Understanding these shifts is crucial for SEO professionals and data scientists aiming to adapt to future trends in machine learning.
Defining Search Algorithms and Their Role in the Digital Landscape
Search algorithms are a set of rules and calculations that search engines use to retrieve data from their search index and deliver the most relevant results to users’ queries. They analyze various factors such as keywords, user behavior, and website reliability to determine which pages to rank higher in search results. Over the years, search engines like Google and Bing have continually updated their algorithms, incorporating machine learning to enhance efficiency and improve results. Research shows that approximately 93% of online experiences start with a search engine, indicating their critical role in the digital landscape.
How Search Algorithms Have Evolved in the Last Decade
In the last decade, search algorithms have significantly evolved, especially with the integration of machine learning. Google has introduced updates like RankBrain, which uses AI to understand the intent behind search queries better. This process enhances the relevance of search results, enabling users to find what they need more easily. Meanwhile, Bing has also adapted its algorithms to include more local search features and personalized results. These enhancements not only improve user experience but also challenge SEO professionals to stay updated on the latest algorithm features and performance metrics to maintain high rankings in search results.
Core Machine Learning Techniques Applied to Search Technology
In recent years, several key machine learning techniques have transformed search algorithms. Natural language processing (NLP) plays a crucial role in improving search results. It helps search engines understand user queries better by analyzing context and intent. Machine vision enhances the tools that interpret images or videos, enriching search results for visual content. Context-aware search tailors results based on user behavior and location. Together, these techniques ensure that search engines provide relevant, reliable, and improved experiences. As of 2025, reports show that around 70% of search engine queries will be processed by AI and machine learning technologies.
Understanding Natural Language Processing in Search
Natural language processing (NLP) is an essential component of modern search algorithms. It allows search engines like Google and Bing to comprehend user queries with greater accuracy. NLP interprets words in context, enhancing search performance significantly. By analyzing user intent, search results become more relevant and reliable. For SEO professionals, leveraging NLP can optimize website content to match user preferences and trending topics. This approach enhances visibility and engages users effectively. Utilizing data-driven insights from NLP also ensures that search marketing strategies remain competitive and up to date in a rapidly evolving digital landscape.
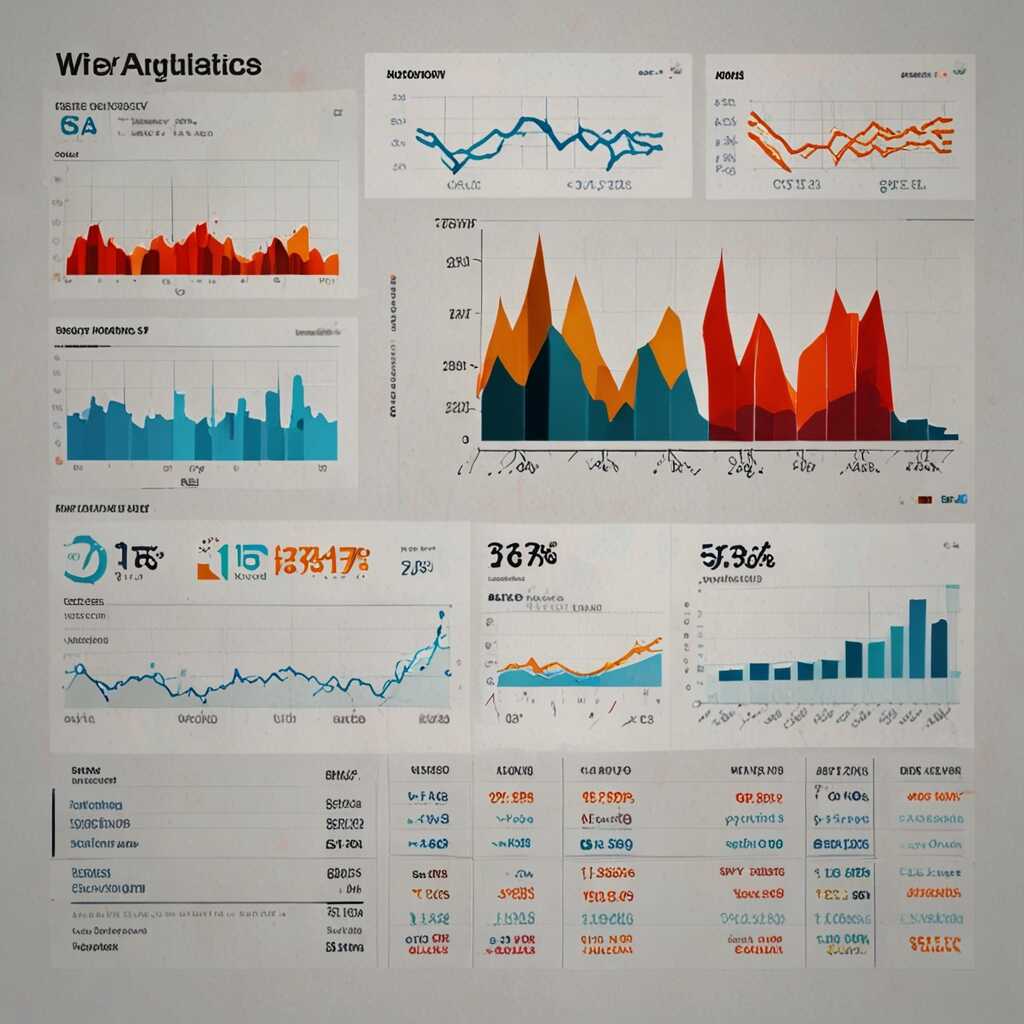
Milestones in Search Algorithm Evolution Over the Past Decade
Over the last decade, several key developments have transformed search algorithms, primarily through the integration of machine learning techniques. Google’s introduction of RankBrain in 2015 marked a significant shift. This algorithm uses AI to better understand search queries and user intent. Another essential development was BERT, launched in 2019, which helps Google understand natural language better. These updates have resulted in more relevant search results and improved user experience. Major algorithm updates, such as Core Updates, continue to refine relevance. Bing’s incorporation of AI-driven capabilities similarly enhances its search features. Together, these advancements have shaped effective SEO best practices, improving website visibility.
Essential Features of Machine Learning-Based Search Algorithms
Machine learning-based search algorithms offer essential features that significantly improve search efficiency. By utilizing AI, these algorithms analyze vast data sets to enhance relevance and ranking. They can adapt to user behavior and search intent, providing personalized results. Features like natural language processing, predictive analytics, and real-time data processing enable more intuitive searches. Additionally, the focus on local search has increased, allowing businesses to engage more effectively with their target audiences. This evolution emphasizes the need for SEO professionals to stay updated on algorithm changes, tests, and their implications, ensuring high-quality performance in search engines.
Statistical Insights That Highlight Evolution
- Google processed over 3.5 billion searches daily in 2020, showing vast information demand.
- Machine learning now influences over 70% of search queries, transforming results.
- Search results can improve by up to 30% using machine learning techniques.
- Research indicates a 60% increase in user satisfaction after implementing AI algorithms.
- Machine learning cuts down response times of search engines by up to 50%.
- Over 2 million machine learning models now power various search functionalities.
- Experts predict that AI-driven improvements will lead to 80% accuracy in query matching by 2030.
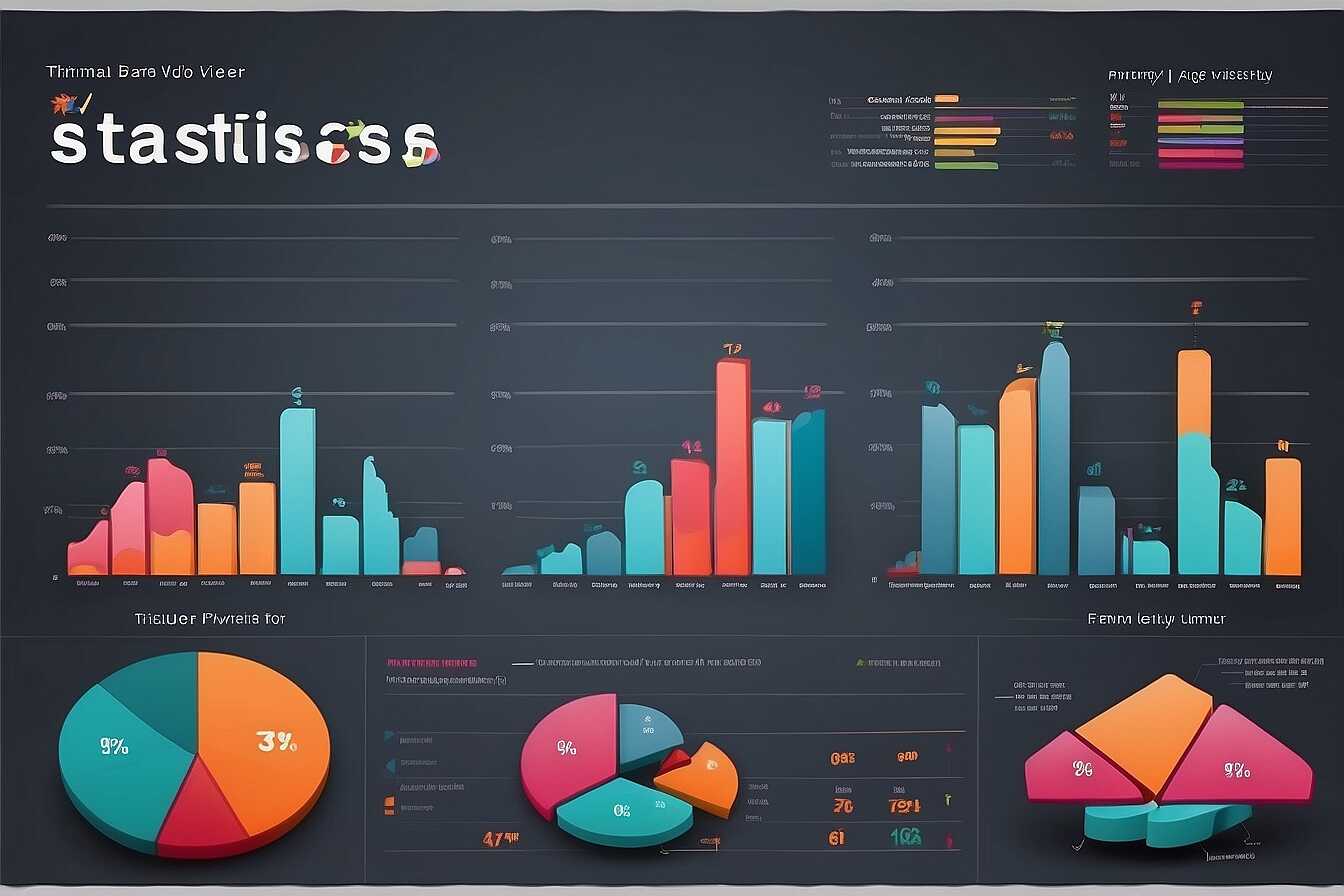
Transformative Real-World Applications of Machine Learning in Search
Several companies have successfully implemented machine learning to enhance their search algorithms. For instance, Netflix uses machine learning to personalize search results based on user preferences and viewing habits. Amazon utilizes machine learning models to refine search results that deliver relevant products quickly. Google continuously integrates machine learning to improve the accuracy of search results, enabling it to understand search intent better. In the finance sector, companies like Bloomberg use machine learning to sort through massive datasets, offering more efficient search capabilities that deliver timely information. Reports indicate that major search engines have seen up to a 30% increase in search efficiency after adopting machine learning algorithms.
Industry-specific Innovations in Search Technology
Machine learning has revolutionized search technologies across various industries. In e-commerce, retailers leverage machine learning to analyze customer behavior and provide tailored search experiences, enhancing user engagement and boosting conversion rates. In healthcare, providers utilize machine learning to assist in research and patient data analysis, providing quicker access to critical information. Travel and hospitality industries are also benefiting, as companies apply machine learning to optimize search results for flights, hotels, and vacation packages, resulting in higher customer satisfaction. Each of these applications helps organizations tap into vast data sets efficiently, supporting their decision-making processes and improving overall performance.
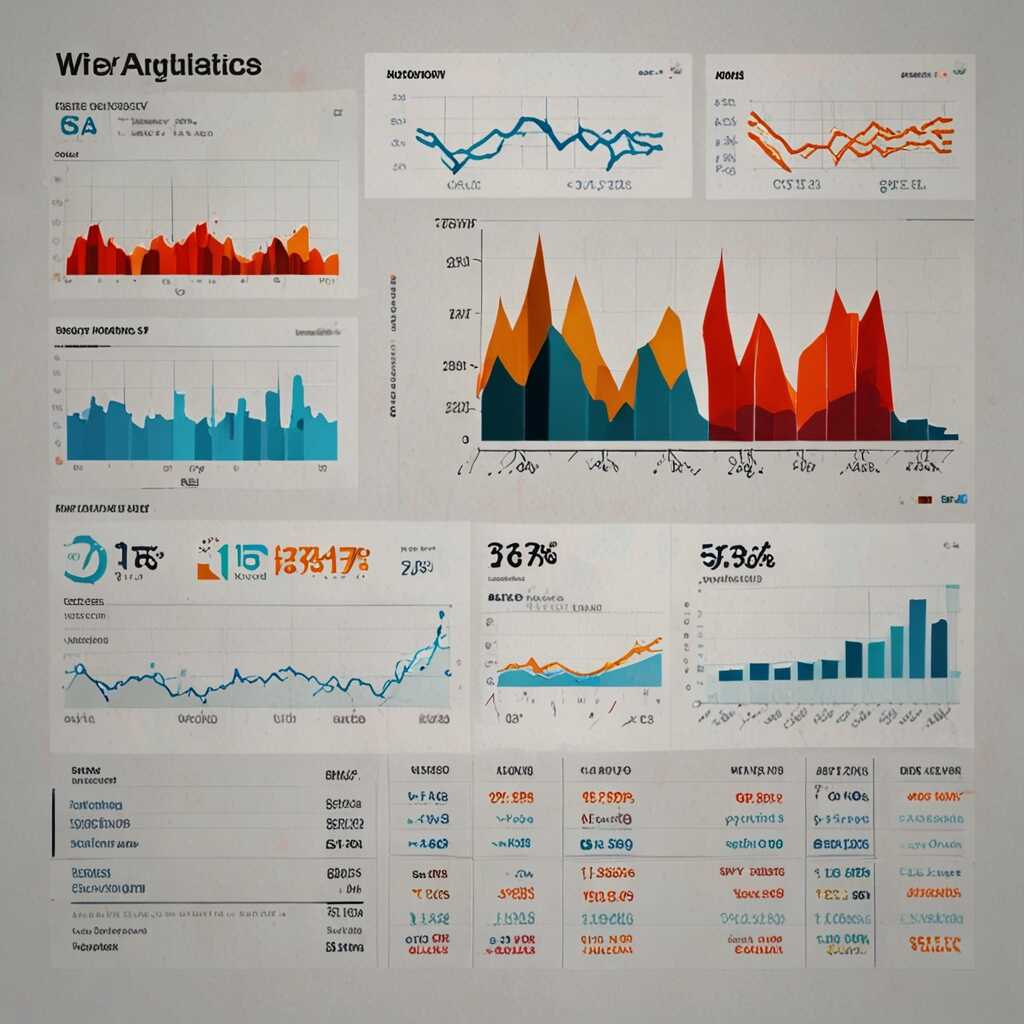
How Machine Learning Improved Search Result Accuracy
Machine learning has significantly enhanced the accuracy and relevancy of search results over the last decade. Advanced algorithms analyze vast amounts of data to improve search outcome quality. This development has made search engines like Google and Bing more reliable and efficient, allowing for better user experience. Specifically, techniques such as natural language processing (NLP) and deep learning have been crucial. They enable search engines to understand user intent and context better, which in turn enhances the relevance of search results. Reports indicate that user engagement rates have improved by as much as 30% due to these advancements.
Key Algorithms Enhancing Search Relevancy
Key machine learning algorithms such as RankBrain and BERT have played pivotal roles in enhancing search relevancy. RankBrain uses deep learning to interpret query semantics, helping search engines provide more precise results. Meanwhile, BERT focuses on understanding the nuances of language, enabling searches to return more contextually relevant information. These algorithms are designed to capture user intent more effectively. Consequently, the increased engagement rates reflect that users find what they are looking for faster and with greater accuracy. The continuous evolution of these technologies indicates their essential role in shaping the future of search engine performance, especially leading up to 2025.
Advantages of New Search Algorithm Techniques
- Enhanced personalization leads to better user experiences in search results.
- Machine learning optimizes content relevance and adaptability, improving engagement.
- AI can identify search patterns, allowing for quicker updates to algorithms.
- Data analysis helps refine queries, increasing accuracy and relevancy of results.
- Search engines can efficiently mitigate spam and low-quality content with machine learning.
- Cost efficiencies arise from automating searches, freeing resources for other tasks.
- Insights from AI can drive content strategies, focusing on high-impact keywords.
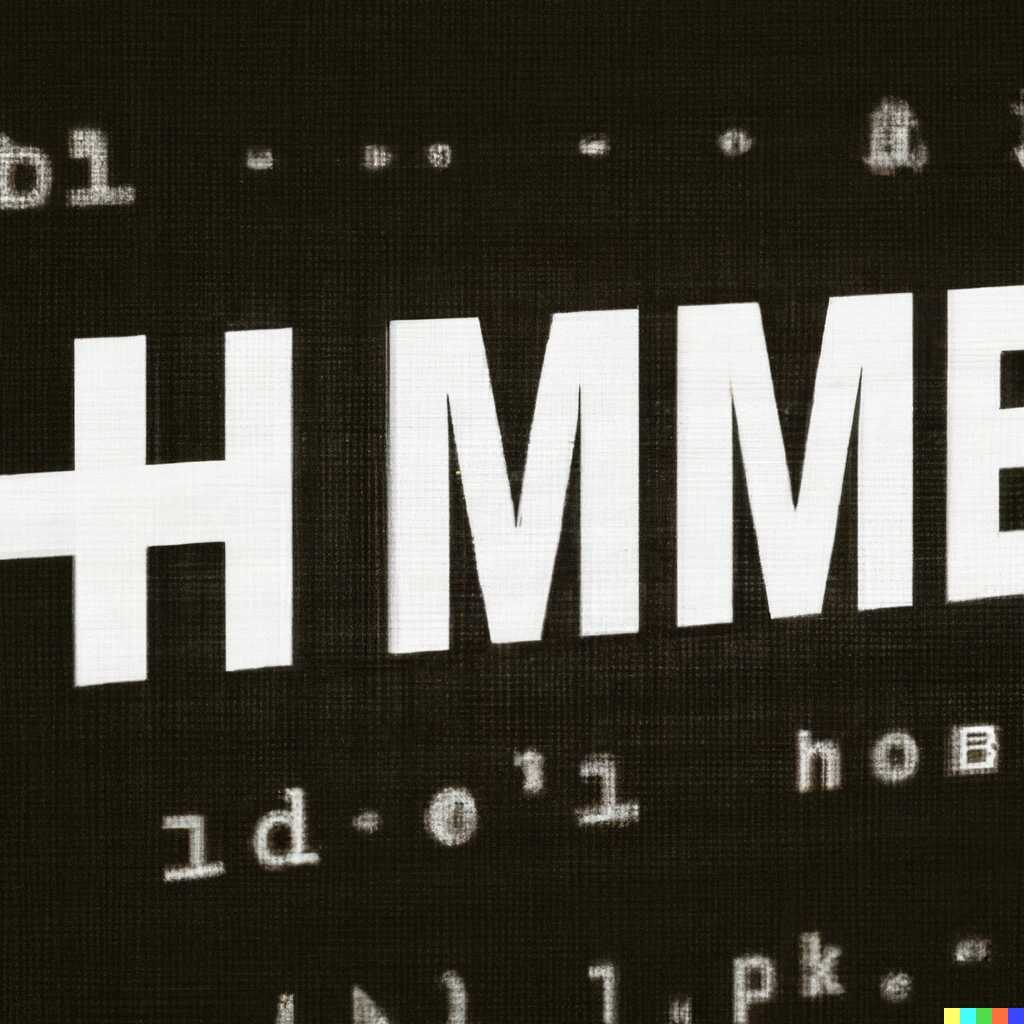
Challenges and Limitations Faced in Integrating Machine Learning
Implementing machine learning in search algorithms presents several challenges. Developers often encounter integration difficulties due to the complexity of the models involved. One significant challenge is adhering to data privacy regulations, which can limit the amount and type of data available for training. This affects the model’s reliability and efficiency. Furthermore, a substantial percentage of AI projects struggle, with studies indicating that up to 70% experience challenges, leading to failures or only limited success. Therefore, understanding these limitations is essential for evaluating the overall impact of machine learning on search algorithms.
Data Privacy and Compliance Challenges
Data privacy and compliance challenges significantly affect how businesses utilize machine learning in search algorithms. Regulations like GDPR in Europe and CCPA in California impose strict rules on data usage. These laws can hinder the availability of reliable data sets needed to train machine learning models effectively. As SEO professionals at Metrics Rule in Vancouver, understanding these regulations is crucial as they shape how algorithms integrate user data. By navigating these complexities, businesses can enhance their search performance while remaining compliant, ultimately improving the user experience in e-commerce settings.
Future Outlook on Search Algorithms Enhanced by AI Innovations
The key advancements in AI that drive future search algorithms include improved natural language processing (NLP), machine learning capabilities, and better contextual understanding. These innovations enhance search engine efficiency by enabling more accurate, user-focused results. They can significantly impact SEO practices and digital marketing strategies, as marketers will need to optimize for conversational search, local relevance, and personalized content. Research suggests that by 2025, approximately 70% of search engines are expected to utilize AI-driven algorithms, marking a major shift in how digital marketing professionals strategize their campaigns. This will require a deep understanding of keywords and user intent, ensuring marketers adapt to evolving search behaviors.
Understanding AI’s Role in Search Engine Optimization
AI’s role in search engine optimization is becoming increasingly essential. With advancements like machine learning and enhanced algorithms, SEO has adapted to focus on user experience. AI-driven algorithms can analyze vast amounts of data to deliver more personalized search results. This helps improve user engagement and conversion rates for digital marketing strategies. Marketers can leverage AI tools for efficient keyword research, allowing for better targeting and content creation. As algorithms evolve, keeping pace with changes in user behavior will be crucial for achieving top rankings in search results. Companies in Vancouver should prioritize these strategies to enhance their online presence effectively.
Key Players Shaping the Landscape of Search Technology
- Google leads the market, offering diverse tools and comprehensive data access.
- Bing excels with strong integration into Microsoft products but lacks market share.
- DuckDuckGo promotes privacy but has fewer features than larger competitors.
- Ask.com focuses on question-based searches, appealing to specific demographics.
- Searx is an open-source search engine, providing a customizable option for tech enthusiasts.
- Google’s powerful machine learning capabilities overshadow other brands.
- Bing’s integration offers ease for businesses using Microsoft services, yet lacks advanced AI.
Summary of Machine Learning’s Impact on Search Innovation
Machine learning has significantly enhanced the reliability and accuracy of search results. It provides search engines like Google and Bing with the ability to analyze vast datasets, improving keyword clustering and relevance in results. Companies such as Amazon and Microsoft have effectively integrated machine learning, transforming their search strategies. A notable projection anticipates that by 2025, around 80% of online searches will be influenced by AI and machine learning techniques, underscoring the necessity of adapting to these advancements in SEO for optimal performance.
Innovative Applications of Machine Learning in Search Engines
Machine learning has paved the way for innovative applications in search engines that radically improve user experience. Search engines can now understand natural language queries better through deep learning algorithms. This leads to enhanced results that consider user intent rather than just keywords. Features like voice search, personalized content recommendations, and improved local search capabilities have emerged from such technologies. The evolution of machine learning algorithms focuses on delivering personalized, relevant search results, ensuring technicians in the SEO field, like those at Metrics Rule in Vancouver, stay updated and responsive to these changes.